What is Generative AI?
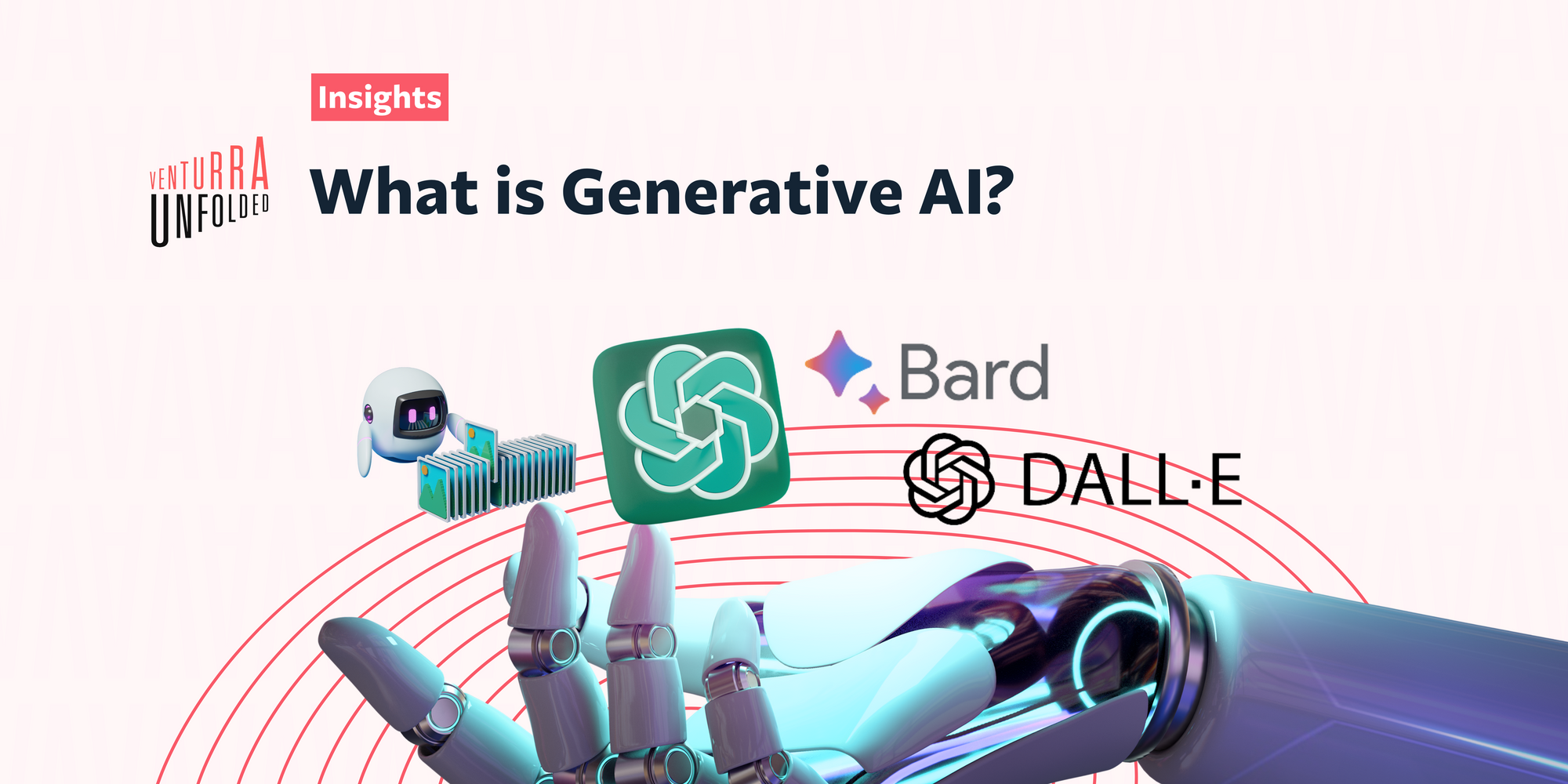
The power of generative AI is undeniable. This form of machine learning allows computers to generate new and exciting content, from music and art to entire virtual worlds. But what does generative AI really mean?
In simple terms, generative AI is a type of machine learning that can create new data. This data can be anything from text to images to music. Generative AI is often used to create realistic and creative content, but it can also be used for more practical purposes, such as generating new product designs or optimizing business processes.
Generative AI is driven by extensive machine learning models known as foundation models (FMs), which are pre-trained on massive datasets. Among these FMs, there is a specialized subset called large language models (LLMs) that undergo training on trillions of words encompassing various natural-language tasks. One of the most popular LLM is ChatGPT. ChatGPT is a chatbot that can generate human-quality text. It can create realistic conversations, write stories, or even generate code.
What's the Difference Between AI and Machine-Learning?
Artificial intelligence (AI) is a broad term that refers to the ability of machines to mimic human intelligence. Machine learning is a type of AI that allows machines to learn from data without being explicitly programmed.
In other words, machine learning is a way of teaching machines to perform tasks without explicitly telling them how to do them. Instead, machines are given data and allowed to learn from it on their own.
This is in contrast to traditional programming, where programmers explicitly tell machines what to do. For example, a programmer might tell a machine to "add two numbers together" or "sort a list of numbers."
Machine learning is becoming increasingly important as the amount of data available to machines grows. With more data, machines can learn to perform more complex tasks. For example, machine learning is being used to develop self-driving cars, fraud detection systems, and recommender systems
What are the Types of Machine-Learning?
Machine learning models come in various types, each built on a foundation of classical statistical techniques dating back to the 18th and 20th centuries, initially designed for small data sets. The pioneers of computing, including the renowned Alan Turing, started working on the fundamental concepts of machine learning during the 1930s and 1940s. However, it was not until the late 1970s that advancements in computer technology made it possible to deploy these techniques outside of laboratories.
See the graphic timeline of AI discovery here
In the past, machine learning primarily focused on predictive models, used to observe and categorize patterns in data, such as recognizing adorable cats in images. The breakthrough came with Generative AI, empowering machine learning to go beyond perception and classification, and enabling it to create images or text descriptions on demand. The advent of Generative AI has opened up new horizons for machine learning, propelling it into the realm of creative expression and limitless possibilities.
How Does the Generative AI Built?
Building a generative AI model is undoubtedly a formidable task, one that has mostly been taken on by well-established tech giants with substantial resources at their disposal. Companies like OpenAI, renowned for creating ChatGPT, former GPT models, and DALL-E, have garnered billions in funding from prominent donors. Similarly, DeepMind, a subsidiary of Alphabet (the parent company of Google), and Meta, have ventured into the realm of generative AI, showcasing products like Make-A-Video. These industry leaders boast teams comprising some of the world's most talented computer scientists and engineers.
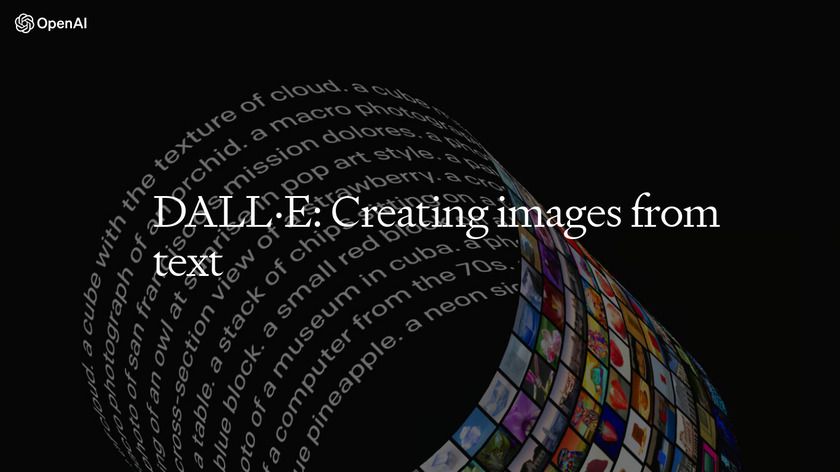
However, it's not just about having talent; the financial investment required is significant. When you embark on training a model that encompasses nearly the entire expanse of the internet, costs inevitably skyrocket. While exact figures from OpenAI are undisclosed, estimations reveal that GPT-3 underwent training on approximately 45 terabytes of text data—equivalent to about one million feet of bookshelf space or a quarter of the entire Library of Congress—at an estimated cost of several million dollars. Needless to say, these are resources beyond the reach of your typical startup company. Nevertheless, as the landscape of technology continues to evolve, we may witness more accessible avenues for startups to harness the power of generative AI in the future.
What challenges can a generative AI model overcome?
Generative AI tools, like ChatGPT, have undoubtedly become sources of endless entertainment, akin to captivating toys. However, their potential extends far beyond amusement, presenting significant opportunities for businesses. By harnessing generative AI, organizations can swiftly generate a diverse range of credible written content, adapt and refine it based on feedback, and tailor it to specific purposes. This transformative capability holds promise for various industries; IT and software organizations can leverage AI's ability to produce instantaneous and mostly accurate code, while marketing teams can tap into generative AI for crafting compelling copy. In essence, any organization seeking to generate clear and impactful written materials can reap the benefits of generative AI. Furthermore, this technology enables the creation of more sophisticated technical materials, such as higher-resolution versions of medical images, ultimately saving valuable time and resources for organizations. The newfound efficiency opens doors to exploring fresh business opportunities and creating even greater value.
While the development of a generative AI model demands substantial resources, rendering it feasible primarily for larger, well-resourced companies, the approach to adopting generative AI is versatile. Businesses have the option to either utilize off-the-shelf generative AI solutions or fine-tune the models to perform specific tasks. For instance, if you require slides tailored to a particular style, the model can "learn" how headlines are typically written based on existing slide data and subsequently generate appropriate headlines when fed with relevant slide information. The adaptability of generative AI empowers organizations to customize its applications to meet their precise needs, further augmenting the technology's potential impact.
What's the verdict for Generative AI?
In conclusion, generative AI is revolutionizing the way businesses approach content creation and problem-solving. With its ability to generate credible and tailored written materials in seconds, this technology holds immense promise for various industries, ranging from IT and software development to marketing and medical imaging. While building a generative AI model requires substantial resources, it has become a viable option primarily for larger companies with significant investments in AI research and development.
Despite the resource-intensive nature of building generative AI models, businesses can still leverage the power of this technology through off-the-shelf solutions or fine-tuning models for specific tasks. The flexibility and versatility of generative AI allow organizations to save time, streamline operations, and explore new business opportunities.
As the field of generative AI continues to evolve, we anticipate even more breakthroughs and applications that will further shape the future of content creation and problem-solving. At Venturra, we remain committed to supporting and investing in innovative startups that harness the potential of generative AI and other cutting-edge technologies. Through these advancements, we aim to drive positive change and empower businesses to thrive in an increasingly dynamic and competitive landscape. Together, we can embrace the possibilities offered by generative AI and unlock a new era of creativity and innovation.